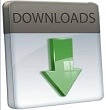

- #WESTERN MICHIGAN UNIVERSITY GAUSSIAN SOFTWARE SERIES#
- #WESTERN MICHIGAN UNIVERSITY GAUSSIAN SOFTWARE FREE#
Schewe, eds., MOS-SIAM Series on Optimization, SIAM, Philadelphia, 2015, pp. Römisch, Chapter 14: Methods for Verifying Booked Capacities, in: Evaluating Gas Network Capacities, Th.

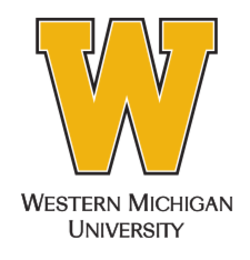
Wegner- Specht, Chapter 13: Empirical Observations and Statistical Analysis of Gas Demand Data, in: Evaluating Gas Network Capacities, Th. Landry, eds., Math for the Digital Factory, 27 of Mathematics in Industry / The European Consortium for Mathematics in Industry, Springer International Publishing AG, Cham, 2017, x+348 pages, (Collection Published), DOI 10.1007/978-7-4. Rantzer, eds., Lecture Notes in Mathematics 2227, Springer Nature Switzerland AG, Cham, 2018, pp. Gasnikov, Chapter 8: Mirror Descent and Convex Optimization Problems with Non-smooth Inequality Constraints, in: Large Scale and Distributed Optimization, P. Then, a stochastic optimization algorithm with much cheaper iteration is used to solve the deterministic problem with high probability.Ī. A deterministic objective function is represented as an expectation of a simple random function. The idea behind this approach is usually called "randomization". Such algorithms, developed for stochastic optimization problems, turn out to be efficient for solving complex deterministic problems. The main mathematical challenge is to obtain non-asymptotic convergence rates for the proposed algorithm. The goal is to develop an algorithm which with high probability gives a good approximation to the minimum of this objective. In another typical problem class, the objective is given as an expectation of a function depending on random parameters. A major research topic at WIAS is therefore the derivation of gradient formulae for probabilistic constraints and the development of algorithmic solution approaches on their basis. This complicates in particular the derivation of important structural properties like convexity or differentiability. The mathematical challenge of these constraints consists in the absence of an explicit formula for the occuring probability functions which can be approximated only with a limited precision.
#WESTERN MICHIGAN UNIVERSITY GAUSSIAN SOFTWARE FREE#
In the context of gas network optimization under random loads, the maximization of free capacities in the nodes under load coverage with given probability plays an important role (see picture right). A typical application is the control of a water reservoir under random inflows and bounds for the water level in the reservoir (see picture left). Here, the decisions guarantee that a given random inequality system (e.g., satisfaction of the random demand of a certain good) is fulfilled at a specified minimum probability. A typical such problem class is defined by so-called probabilistic (or chance) constraints. The solution of such problems aims in general at finding cost optimal decisions which at the same time are robust against the effect of randomness. Stochastic Optimization in the widest sense is concerned with optimization problems influenced by random parameters in the objective or constraints. Quantitative Analysis of Stochastic and Rough Systems.Probabilistic Methods for Dynamic Communication Networks.Numerical Methods for Innovative Semiconductor Devices.Modeling, Analysis, and Scaling Limits for Bulk-Interface Processes.Nonsmooth Variational Problems and Operator Equations.Thermodynamic Modeling and Analysis of Phase Transitions.Stochastic Algorithms and Nonparametric Statistics.Nonlinear Optimization and Inverse Problems.Numerical Mathematics and Scientific Computing.Optimization and Control in Technology and Economy.Conversion, Storage and Distribution of Energy.Mathematical research topic of WIAS - Stochastic Optimization
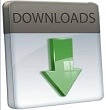